Data Science: 4 valuable applications in finance
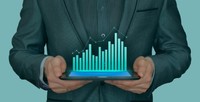
Long before data science came into use, data was still a mainstay in finance. However, data collection and processing in the traditional setting involved complex, lengthy, and inaccurate processes that are nothing close to today's standards.
Because of this relationship between data and finance, the financial sector is among the industries that have the most applications for data science. This post takes a deep look at data science and its valuable application in the finance sector.
Data Science in Finance
Data science is the scientific discipline of making sense of complex, large-scale data to develop actionable insights. It enables businesses to focus on the insights that help decision-makers make better financial decisions.
While making sense of your business's data is an excellent idea, it can be challenging and requires hiring someone skilled in financial data science. Alternatively, you may want to outsource your data analysis to a data science consulting agency such as RTSLabs.com, which offers a mix of experience and affordability.
Applications of Data Science in Finance
1. Risk Analytics
Taking risks is part of business, and there is no way of eliminating all the risks. However, it is possible to minimize risks to the lowest possible levels with the right insights from collected data. For example, data collected over time can provide insights into where a business went into loss, why, the impact, and the number of times losses occurred under the same circumstances.
With this kind of insight, a business owner or manager can develop ways of mitigating the loss in the future. If you are leading a lending institution, data science can help you analyze the trustworthiness of your customers by analyzing data on their past transactions and general information, which can help determine the risk associated with the customers you lend to.
2. Real-time Analytics
Traditionally data engineers relied on old data as old as several weeks, months, or even more. The problem with this data is that it doesn't allow for real-time response to emerging needs. Thankfully, today's data analysis tools allow real-time access to insights necessary for growth.
Real-time data analysis ensures that decision-makers in an organization can make decisions based on insights derived from real-time data—for example, consumer transactions, credit scores, and other necessary decision-advising behaviors.
3. Predictive Consumer Analytics
Consumer personalization is a big deal in any business. It involves collecting a consumer's data and making sense of it to create a consumer persona whose behavior you can predict. Having such insight helps you package your communication to relate to them based on where they stand in the buyer's journey.
Predictive consumer analytics can also help predict when a consumer may be contemplating switching to a competitor allowing you to respond with suitable offers to encourage them to stay. Additionally, it is beneficial in identifying and eliminating below-zero customers that may push your marketing cost upwards.
4. Fraud Detection
Fraud has always been and is still one of the biggest concerns in the financial sector. The most rampant types of fraud include credit card fraud, insurance fraud, and identity theft. Traditionally, the inefficiencies in data analysis tools meant that companies could only identify fraud long after the damage had happened.
Advances in data science have provided financial institutions with real-time insights to help detect and act against such crimes before they happen. Even when they do occur, the chances of detecting anomalies are significantly high, allowing an institution to stop the crime before it causes a lot of damage. For example, unusual credit card usage or bank transactions can trigger a fraud alert and stop it before it inflicts much damage.